目錄
1. 模擬退火算法實現步驟?
2. python實現
3. 實驗結果
4. 參考文獻
模擬退火算法的基本原理在這里就不一一贅述了, 關于原理,可以參考百度百科、博客1、博客2
在本節按照基本實現步驟實現模擬退火算法, 對于模擬退火算法的高級封裝(類封裝), 可以參考模擬退火算法之特征選擇的python實現(二)
1. 模擬退火算法實現步驟?
2. python實現
import numpy as np
from sklearn.preprocessing import StandardScaler
from sklearn.neural_network import MLPRegressor
from sklearn.metrics import mean_squared_error
import scipy.io as sio
from sklearn.model_selection import train_test_split
def get_data_subset(x_data, columns):
return x_data[:, columns]
def get_initial_solution(feature_size, selected_feature):
sol = np.arange(feature_size-1)
np.random.shuffle(sol)
return sol[:selected_feature]
def get_neighbor(current_solution, feature_size, temperature):
all_features = range(feature_size-1)
selected = current_solution
not_selected = np.setdiff1d(all_features, selected)
# swap one selected feature with one non-selected feature
num_swaps = int(min(np.ceil(np.abs(np.random.normal(0, 0.1*len(selected)*temperature))), np.ceil(0.1*len(selected))))
feature_out = np.random.randint(0, len(selected), num_swaps) # 產生num_swaps個樣本索引(從range(len(selected))中)
selected = np.delete(selected, feature_out)
feature_in = np.random.randint(0, len(not_selected), num_swaps) # 產生num_swaps個樣本索引(從range(len(not_selected))中)
selected = np.append(selected, not_selected[feature_in])
return selected
def get_cost(solution, x_train, x_test, y_train, y_test):
x_scale, y_scale = StandardScaler(), StandardScaler()
x_train_scaled = x_scale.fit_transform(x_train)
x_test_scaled = x_scale.transform(x_test)
y_train_scaled = y_scale.fit_transform(y_train.reshape(-1, 1))
y_test_scaled = y_scale.transform(y_test.reshape(-1, 1))
limited_train_data = get_data_subset(x_train_scaled, solution)
limited_test_data = get_data_subset(x_test_scaled, solution)
model = MLPRegressor(hidden_layer_sizes=43)
model.fit(limited_train_data, y_train_scaled.ravel())
y_test_pred = model.predict(limited_test_data)
y_test_pred = y_scale.inverse_transform(y_test_pred)
return round(mean_squared_error(y_test, y_test_pred), 4)
def get_probability(temperature, delta_cost):
return np.exp(delta_cost/temperature)
def simulated_annealing(initT, minT, alpha, iterations, features, x_train, x_test, y_train, y_test):
temperature = initT # 當前溫度
solution = get_initial_solution(feature_size=features_size, selected_feature=sel_feature)
cost = get_cost(solution, x_train, x_test, y_train, y_test)
temp_history = [temperature]
best_cost_history = []
best_solution_history = []
best_cost = cost
best_solution = solution
while temperature > minT:
for k in range(iterations):
next_solution = get_neighbor(solution, features, temperature)
next_cost = get_cost(next_solution, x_train, x_test, y_train, y_test)
probability = 0
if next_cost > cost: # 計算向差方向移動的概率 (即移動后的解比當前解要差)
probability = get_probability(temperature, cost-next_cost)
if next_cost < cost or np.random.random() < probability: # 朝著最優解移動或以一定概率向差方向移動
cost = next_cost
solution = next_solution
if next_cost < best_cost: # 最優值和最優解
best_cost = cost
best_solution = solution
print("當前溫度:", round(temperature, 2))
print("當前溫度下最好的得分:", best_cost)
print("當前溫度下波長數量:", len(solution))
temperature *= alpha
temp_history.append(temperature)
best_cost_history.append(best_cost)
best_solution_history.append(best_solution)
return temp_history, best_cost_history, best_solution_history
# 1.數據獲取
mat = sio.loadmat('NDFNDF_smote.mat')
data = mat['NDFNDF_smote']
x, y = data[:, :50], data[:, 50]
print('原始數據大小:', x.shape, y.shape)
# 2.樣本集劃分和預處理
x_train, x_test, y_train, y_test = train_test_split(x, y, test_size=0.2, random_state=1)
features_size = x.shape[1]
sel_feature = 5
temp_his, best_cost_his, best_solution_his = simulated_annealing(initT=100,
minT=1,
alpha=0.95,
iterations=50,
features=features_size,
x_train=x_train,
x_test=x_test,
y_train=y_train,
y_test=y_test)
3. 實驗結果
4. 參考文獻
謝云.? 模擬退火算法的原理及實現 . 1999. PhD Thesis.
陳華根, 吳健生, 王家林, & 陳冰. (2004).? 模擬退火算法機理研究 ?(Doctoral dissertation).
更多文章、技術交流、商務合作、聯系博主
微信掃碼或搜索:z360901061
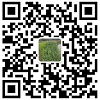
微信掃一掃加我為好友
QQ號聯系: 360901061
您的支持是博主寫作最大的動力,如果您喜歡我的文章,感覺我的文章對您有幫助,請用微信掃描下面二維碼支持博主2元、5元、10元、20元等您想捐的金額吧,狠狠點擊下面給點支持吧,站長非常感激您!手機微信長按不能支付解決辦法:請將微信支付二維碼保存到相冊,切換到微信,然后點擊微信右上角掃一掃功能,選擇支付二維碼完成支付。
【本文對您有幫助就好】元
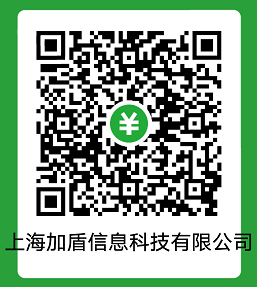