其實像感知機這些基本的機器學習算法,原理自己也都懂,但是還是會在看代碼的時候感到困惑,說不上哪里困惑,但就是困惑!所以,做一些筆記讓自己更清楚一些。
1.
import numpy as np
import matplotlib.pyplot as plt#導入matplotlib庫
from sklearn.datasets import make_blobs
from sklearn.model_selection import train_test_split
np.random.seed(123)
% matplotlib inline
sklearn庫對數據集進行劃分需要使用model_selection函數,該函數的 train_test_split是交叉驗證中常用的函數,功能是從樣本中隨機按比例選取train-data和test-data.
2. DataSet
X, y = make_blobs(n_samples=1000, centers=2)
fig = plt.figure(figsize=(8,6))
plt.scatter(X[:,0], X[:,1], c=y)#畫散點圖
plt.title("Dataset")#設置標題
plt.xlabel("First feature")#設置x軸標簽
plt.ylabel("Second feature")#設置y軸標簽
plt.show()#顯示所畫的圖
(1)plt.figure:
在繪圖過程中,調用figure創建一個繪圖對象,并且使它成為當前的繪圖對象。
(2)make_blobs:
scikit中的make_blobs方法常被用來生成聚類算法的測試數據,直觀地說,make_blobs會根據用戶指定的特征數量、中心點數量、范圍等來生成幾類數據,這些數據可用于測試聚類算法的效果。
- n_samples是待生成的樣本的總數。
- n_features是每個樣本的特征數。
- centers表示類別數
(3) plt.figure(figsize=(8,6))
- figsize:指定figure的寬和高,單位為英寸;
3.
y_true = y[:, np.newaxis]
X_train, X_test, y_train, y_test = train_test_split(X, y_true)
print(f'Shape X_train: {X_train.shape}')
print(f'Shape y_train: {y_train.shape})')
print(f'Shape X_test: {X_test.shape}')
print(f'Shape y_test: {y_test.shape}')
結果:
Shape X_train: (750, 2)
Shape y_train: (750, 1))
Shape X_test: (250, 2)
Shape y_test: (250, 1)
train_test_split是劃分數據集的一個函數。
4. Perceptron model class
class Perceptron():
def __init__(self):
pass
def train(self, X, y, learning_rate=0.05, n_iters=100):
n_samples, n_features = X.shape
# Step 0: Initialize the parameters
self.weights = np.zeros((n_features,1))
self.bias = 0
for i in range(n_iters):
# Step 1: Compute the activation
a = np.dot(X, self.weights) + self.bias
# Step 2: Compute the output
y_predict = self.step_function(a)
# Step 3: Compute weight updates
delta_w = learning_rate * np.dot(X.T, (y - y_predict))
delta_b = learning_rate * np.sum(y - y_predict)
# Step 4: Update the parameters
self.weights += delta_w
self.bias += delta_b
return self.weights, self.bias
def step_function(self, x):
return np.array([1 if elem >= 0 else 0 for elem in x])[:, np.newaxis]
def predict(self, X):
a = np.dot(X, self.weights) + self.bias
return self.step_function(a)
5. Initialization and training the model
p = Perceptron()
w_trained, b_trained = p.train(X_train, y_train,learning_rate=0.05, n_iters=500)
6. Testing
y_p_train = p.predict(X_train)
y_p_test = p.predict(X_test)
print(f"training accuracy: {100 - np.mean(np.abs(y_p_train - y_train)) * 100}%")
print(f"test accuracy: {100 - np.mean(np.abs(y_p_test - y_test)) * 100}%")
7. Visualize decision boundary
def plot_hyperplane(X, y, weights, bias):
"""
Plots the dataset and the estimated decision hyperplane
"""
slope = - weights[0]/weights[1]
intercept = - bias/weights[1]
x_hyperplane = np.linspace(-10,10,10)
y_hyperplane = slope * x_hyperplane + intercept
fig = plt.figure(figsize=(8,6))
plt.scatter(X[:,0], X[:,1], c=y)
plt.plot(x_hyperplane, y_hyperplane, '-')
plt.title("Dataset and fitted decision hyperplane")
plt.xlabel("First feature")
plt.ylabel("Second feature")
plt.show()
8.
plot_hyperplane(X, y, w_trained, b_trained)
更多文章、技術交流、商務合作、聯系博主
微信掃碼或搜索:z360901061
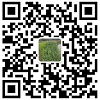
微信掃一掃加我為好友
QQ號聯系: 360901061
您的支持是博主寫作最大的動力,如果您喜歡我的文章,感覺我的文章對您有幫助,請用微信掃描下面二維碼支持博主2元、5元、10元、20元等您想捐的金額吧,狠狠點擊下面給點支持吧,站長非常感激您!手機微信長按不能支付解決辦法:請將微信支付二維碼保存到相冊,切換到微信,然后點擊微信右上角掃一掃功能,選擇支付二維碼完成支付。
【本文對您有幫助就好】元
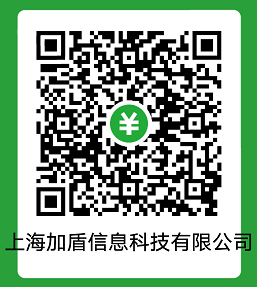