- Apriori 代碼
import pandas as pd
#自定義連接函數,用于實現L_{k-1}到C_k的連接
def connect_string(x, ms):
"""
x:頻繁項集列表
ms: 連接符,這里用 ‘---’
return: 返回長度+1的頻繁項集,即L_{k-1}到C_k的連接
"""
x = list(map(lambda i:sorted(i.split(ms)), x)) #去除序列中的連接符,并將元素排列
n = len(x[0]) #每個頻繁項集的長度為 n
r = []
for i in range(len(x)):
for j in range(i,len(x)): # 遍歷每個元素,并與后面所有元素一一比對
if x[i][:n-1] == x[j][:n-1] and x[i][n-1] != x[j][n-1]:# 當兩個序列的前 n-1 項相同且第 n 項不同
r.append(x[i][:n-1]+sorted([x[j][n-1],x[i][n-1]])) # 將兩個序列不同的第 n 項和前 n-1 項拼接
return r
#尋找關聯規則的函數
def find_rule(d, support, confidence, ms = u'---'):
"""
d:轉換后的0-1數據矩陣
"""
result = pd.DataFrame(index=['support', 'confidence']) #定義輸出結果
support_series = 1.0*d.sum()/len(d) #支持度序列
column = list(support_series[support_series > support].index) #初步根據支持度篩選,得一項頻繁集
k = 0
while len(column) > 1:
k = k+1
print(u'\n正在進行第%s次搜索...' %k)
column = connect_string(column, ms) # 獲取新的頻繁項集
print(u'數目:%s...' %len(column))
sf = lambda i: d[i].prod(axis=1, numeric_only = True) #新一批支持度的計算函數
# DataFrame.prod() 計算各軸的乘積,axis=1計算橫軸
# i 為項集列表,形如:[a,c,e],計算三列的橫向乘積,結果為‘1’表示該項集出現一次
#創建連接數據,這一步耗時、耗內存最嚴重。當數據集較大時,可以考慮并行運算優化。
d_2 = pd.DataFrame(list(map(sf,column)), index = [ms.join(i) for i in column]).T
support_series_2 = 1.0*d_2[[ms.join(i) for i in column]].sum()/len(d) #計算連接后的支持度
column = list(support_series_2[support_series_2 > support].index) #新一輪支持度篩選
support_series = support_series.append(support_series_2)
column2 = []
for i in column: #遍歷可能的推理,如{A,B,C}究竟是A+B-->C還是B+C-->A還是C+A-->B?
i = i.split(ms)
for j in range(len(i)):
column2.append(i[:j]+i[j+1:]+i[j:j+1])
cofidence_series = pd.Series(index=[ms.join(i) for i in column2]) #定義置信度序列
for i in column2: #計算置信度序列
cofidence_series[ms.join(i)] = support_series[ms.join(sorted(i))]/support_series[ms.join(i[:len(i)-1])]
for i in cofidence_series[cofidence_series > confidence].index: #置信度篩選
result[i] = 0.0 # 建新列
result[i]['confidence'] = cofidence_series[i]
result[i]['support'] = support_series[ms.join(sorted(i.split(ms)))]
result = result.sort_index(ascending=False) #結果整理,輸出
print(u'\n結果為:')
print(result.T)
return result.T
- 使用代碼
import pandas as pd
from apriori import * #導入自行編寫的apriori函數
inputfile = '../data/menu_orders.xls'
outputfile = '../tmp/apriori_rules.xls' #結果文件
data = pd.read_excel(inputfile, header = None)
print(u'\n轉換原始數據至0-1矩陣...')
ct = lambda x : pd.Series(1, index = x[pd.notnull(x)]) #轉換0-1矩陣的過渡函數,非空值轉換成‘1’
b = map(ct, data.values) #用map方式執行
data = pd.DataFrame(list(b)).fillna(0) #實現矩陣轉換,空值用0填充
print(u'\n轉換完畢。')
del b #刪除中間變量b,節省內存
support = 0.2 #最小支持度
confidence = 0.5 #最小置信度
ms = '---' #連接符,默認'--',用來區分不同元素,如A--B。需要保證原始表格中不含有該字符
find_rule(data, support, confidence, ms).to_excel(outputfile) #保存結果
更多文章、技術交流、商務合作、聯系博主
微信掃碼或搜索:z360901061
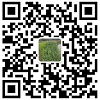
微信掃一掃加我為好友
QQ號聯系: 360901061
您的支持是博主寫作最大的動力,如果您喜歡我的文章,感覺我的文章對您有幫助,請用微信掃描下面二維碼支持博主2元、5元、10元、20元等您想捐的金額吧,狠狠點擊下面給點支持吧,站長非常感激您!手機微信長按不能支付解決辦法:請將微信支付二維碼保存到相冊,切換到微信,然后點擊微信右上角掃一掃功能,選擇支付二維碼完成支付。
【本文對您有幫助就好】元
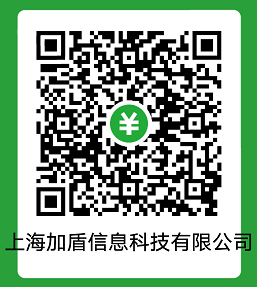