前言
優(yōu)化隨機(jī)森林算法,正確率提高1%~5%(已經(jīng)有90%+的正確率,再調(diào)高會(huì)導(dǎo)致過(guò)擬合)
論文當(dāng)然是參考的,畢竟出現(xiàn)早的算法都被人研究爛了,什么優(yōu)化基本都做過(guò)。而人類最高明之處就是懂得利用前人總結(jié)的經(jīng)驗(yàn)和制造的工具(說(shuō)了這么多就是為偷懶找借口。hhhh)
優(yōu)化思路
1. 計(jì)算傳統(tǒng)模型準(zhǔn)確率
2. 計(jì)算設(shè)定樹(shù)木顆數(shù)時(shí)最佳樹(shù)深度,以最佳深度重新生成隨機(jī)森林
3. 計(jì)算新生成森林中每棵樹(shù)的AUC,選取AUC靠前的一定百分比的樹(shù)
4. 通過(guò)計(jì)算各個(gè)樹(shù)的數(shù)據(jù)相似度,排除相似度超過(guò)設(shè)定值且AUC較小的樹(shù)
5. 計(jì)算最終的準(zhǔn)確率
主要代碼粘貼如下 (注釋比較詳細(xì),就不介紹代碼了)
#-*- coding: utf-8 -*- import time from csv import reader from random import randint from random import seed import numpy as np from numpy import mat from group_11 import caculateAUC_1, plotTree # 建立一棵CART樹(shù) '''試探分枝''' def data_split(index, value, dataset): left, right = list(), list() for row in dataset: if row[index] < value: left.append(row) else: right.append(row) return left, right '''計(jì)算基尼指數(shù)''' def calc_gini(groups, class_values): gini = 0.0 total_size = 0 for group in groups: total_size += len(group) for group in groups: size = len(group) if size == 0: continue for class_value in class_values: proportion = [row[-1] for row in group].count(class_value) / float(size) gini += (size / float(total_size)) * (proportion * (1.0 - proportion))# 二分類執(zhí)行兩次,相當(dāng)于*2 return gini '''找最佳分叉點(diǎn)''' def get_split(dataset, n_features): class_values = list(set(row[-1] for row in dataset))# 類別標(biāo)簽集合 b_index, b_value, b_score, b_groups = 999, 999, 999, None # 隨機(jī)選取特征子集,包含n_features個(gè)特征 features = list() while len(features) < n_features: # 隨機(jī)選取特征 # 特征索引 index = randint(0, len(dataset[0]) - 2) # 往features添加n_features個(gè)特征(n_feature等于特征數(shù)的根號(hào)),特征索引從dataset中隨機(jī)取 if index not in features: features.append(index) for index in features: # 對(duì)每一個(gè)特征 # 計(jì)算Gini指數(shù) for row in dataset: # 按照每個(gè)記錄的該特征的取值劃分成兩個(gè)子集,計(jì)算對(duì)于的Gini(D,A),取最小的 groups = data_split(index, row[index], dataset) gini = calc_gini(groups, class_values) if gini < b_score: b_index, b_value, b_score, b_groups = index, row[index], gini, groups return {'index': b_index, 'value': b_value, 'groups': b_groups} # 每個(gè)節(jié)點(diǎn)由字典組成 '''多數(shù)表決''' def to_terminal(group): outcomes = [row[-1] for row in group] return max(set(outcomes), key=outcomes.count) '''分枝''' def split(node, max_depth, min_size, n_features, depth): left, right = node['groups'] # 自動(dòng)分包/切片 del (node['groups']) if not left or not right: # left或者right為空時(shí) node['left'] = node['right'] = to_terminal(left + right) # 葉節(jié)點(diǎn)不好理解 return if depth >= max_depth: node['left'], node['right'] = to_terminal(left), to_terminal(right) return # 左子樹(shù) if len(left) <= min_size: node['left'] = to_terminal(left) else: node['left'] = get_split(left, n_features) split(node['left'], max_depth, min_size, n_features, depth + 1) # 右子樹(shù) if len(right) <= min_size: # min_size最小的的分枝樣本數(shù) node['right'] = to_terminal(right) else: node['right'] = get_split(right, n_features) split(node['right'], max_depth, min_size, n_features, depth + 1) '''建立一棵樹(shù)''' def build_one_tree(train, max_depth, min_size, n_features): # 尋找最佳分裂點(diǎn)作為根節(jié)點(diǎn) root = get_split(train, n_features) split(root, max_depth, min_size, n_features, 1) return root '''用森林里的一棵樹(shù)來(lái)預(yù)測(cè)''' def predict(node, row): if row[node['index']] < node['value']: if isinstance(node['left'], dict): return predict(node['left'], row) else: return node['left'] else: if isinstance(node['right'], dict): return predict(node['right'], row) else: return node['right'] # 隨機(jī)森林類 class randomForest: def __init__(self,trees_num, max_depth, leaf_min_size, sample_ratio, feature_ratio): self.trees_num = trees_num # 森林的樹(shù)的數(shù)目 self.max_depth = max_depth # 樹(shù)深 self.leaf_min_size = leaf_min_size # 建立樹(shù)時(shí),停止的分枝樣本最小數(shù)目 self.samples_split_ratio = sample_ratio # 采樣,創(chuàng)建子集的比例(行采樣) self.feature_ratio = feature_ratio # 特征比例(列采樣) self.trees = list() # 森林 '''有放回的采樣,創(chuàng)建數(shù)據(jù)子集''' def sample_split(self, dataset): sample = list() n_sample = round(len(dataset) * self.samples_split_ratio) #每棵樹(shù)的采樣數(shù) while len(sample) < n_sample: index = randint(0, len(dataset) - 2) #隨機(jī)有放回的采樣 sample.append(dataset[index]) return sample ##############***Out-of-Bag***################################ # 進(jìn)行袋外估計(jì)等相關(guān)函數(shù)的實(shí)現(xiàn),需要注意并不是每個(gè)樣本都可能出現(xiàn)在隨機(jī)森林的袋外數(shù)據(jù)中 # 因此進(jìn)行oob估計(jì)時(shí)需要注意估計(jì)樣本的數(shù)量 def OOB(self, oobdata, train, trees): '''輸入為:袋外數(shù)據(jù)dict,訓(xùn)練集,tree_list return oob準(zhǔn)確率''' n_rows = [] count = 0 n_trees = len(trees) # 森林中樹(shù)的棵樹(shù) for key, item in oobdata.items(): n_rows.append(item) # print(len(n_rows)) # 所有trees中的oob數(shù)據(jù)的合集 n_rows_list = sum(n_rows, []) unique_list = [] for l1 in n_rows_list: # 從oob合集中計(jì)算獨(dú)立樣本數(shù)量 if l1 not in unique_list: unique_list.append(l1) n = len(unique_list) # print(n) # 對(duì)訓(xùn)練集中的每個(gè)數(shù)據(jù),進(jìn)行遍歷,尋找其作為oob數(shù)據(jù)時(shí)的所有trees,并進(jìn)行多數(shù)投票 for row in train: pre = [] for i in range(n_trees): if row not in oobdata[i]: # print('row: ',row) # print('trees[i]: ', trees[i]) pre.append(predict(trees[i], row)) if len(pre) > 0: label = max(set(pre), key=pre.count) if label == row[-1]: count += 1 return (float(count) / n) * 100 '''建立隨機(jī)森林''' def build_randomforest(self, train): temp_flag = 0 max_depth = self.max_depth # 樹(shù)深 min_size = self.leaf_min_size # 建立樹(shù)時(shí),停止的分枝樣本最小數(shù)目 n_trees = self.trees_num # 森林的樹(shù)的數(shù)目 n_features = int(self.feature_ratio * (len(train[0])-1)) #列采樣,從M個(gè)feature中,選擇m個(gè)(m<samerate): # 將對(duì)比樹(shù)置空 newforest[k] = None result_forest = list() for i in range(0, newforest.__len__()): if not newforest[i] == None: result_forest.append(newforest[i]) return result_forest '''auc優(yōu)化method''' def auc_optimization(auclist,trees_num,trees): # 為auc排序,獲取從大到小的與trees相對(duì)應(yīng)的索引列表 b = sorted(enumerate(auclist), key=lambda x: x[1], reverse=True) index_list = [x[0] for x in b] auc_num = int(trees_num * 2 / 3) # 取auc高的前auc_num個(gè) print('auc: ', auc_num, index_list) newTempForest = list() for i in range(auc_num): # myRF.trees.append(tempForest[i]) # newTempForest.append(myRF.trees[index_list[i]]) newTempForest.append(trees[index_list[i]]) return newTempForest '''得到森林中決策樹(shù)的最佳深度''' def getBestDepth(min_size,sample_ratio,trees_num,feature_ratio,traindata,testdata): max_depth = np.linspace(1, 15, 15, endpoint=True) # max_depth=[5,6,7,8,9,10,11,12,13,14,15] scores_final = [] i=0 for depth in max_depth: # 初始化隨機(jī)森林 # print('=========>',i,'<=============') myRF_ = randomForest(trees_num, depth, min_size, sample_ratio, feature_ratio) # 生成隨機(jī)森林 myRF_.build_randomforest(traindata) # 測(cè)試評(píng)估 acc = myRF_.accuracy_metric(testdata[:-1]) # print('模型準(zhǔn)確率:', acc, '%') # scores_final.append(acc.mean()) scores_final.append(acc*0.01) i=i+1 # print('scores_final: ',scores_final) # 找到深度小且準(zhǔn)確率高的值 best_depth = 0 temp_score = 0 for i in range(len(scores_final)): if scores_final[i] > temp_score: temp_score = scores_final[i] best_depth = max_depth[i] # print('best_depth:',np.mean(scores_final),best_depth) # plt.plot(max_depth, scores_final, 'r-', lw=2) # # plt.plot(max_depth, list(range(0,max(scores_final))), 'r-', lw=2) # plt.xlabel('max_depth') # plt.ylabel('CV scores') # plt.ylim(bottom=0.0,top=1.0) # plt.grid() # plt.show() return best_depth '''對(duì)比不同樹(shù)個(gè)數(shù)時(shí)的模型正確率''' def getMyRFAcclist(treenum_list): seed(1) # 每一次執(zhí)行本文件時(shí)都能產(chǎn)生同一個(gè)隨機(jī)數(shù) filename = 'DataSet3.csv' #SMOTE處理過(guò)的數(shù)據(jù) min_size = 1 sample_ratio = 1 feature_ratio = 0.3 # 盡可能小,但是要保證 int(self.feature_ratio * (len(train[0])-1)) 大于1 same_value = 20 # 向量?jī)?nèi)積的差(小于此值認(rèn)為相似) same_rate = 0.63 # 樹(shù)的相似度(大于此值認(rèn)為相似) # 加載數(shù)據(jù) dataset, features = load_csv(filename) traindata, testdata = split_train_test(dataset, feature_ratio) # 森林中不同樹(shù)個(gè)數(shù)的對(duì)比 # treenum_list = [20, 30, 40, 50, 60] acc_num_list = list() acc_list=list() for trees_num in treenum_list: # 優(yōu)化1-獲取最優(yōu)深度 max_depth = getBestDepth(min_size, sample_ratio, trees_num, feature_ratio, traindata, testdata) print('max_depth is ', max_depth) # 初始化隨機(jī)森林 myRF = randomForest(trees_num, max_depth, min_size, sample_ratio, feature_ratio) # 生成隨機(jī)森林 myRF.build_randomforest(traindata) print('Tree_number: ', myRF.trees.__len__()) # 計(jì)算森林中每棵樹(shù)的AUC auc_list = caculateAUC_1.caculateRFAUC(testdata, myRF.trees) # 選取AUC高的決策數(shù)形成新的森林(auc優(yōu)化) newTempForest = auc_optimization(auc_list,trees_num,myRF.trees) # 相似度優(yōu)化 myRF.trees = similarity_optimization(newTempForest, same_value, same_rate) # 測(cè)試評(píng)估 acc = myRF.accuracy_metric(testdata[:-1]) print('myRF1_模型準(zhǔn)確率:', acc, '%') acc_num_list.append([myRF.trees.__len__(), acc]) acc_list.append(acc) print('trees_num from 20 to 60: ', acc_num_list) return acc_list if __name__ == '__main__': start = time.clock() seed(1) # 每一次執(zhí)行本文件時(shí)都能產(chǎn)生同一個(gè)隨機(jī)數(shù) filename = 'DataSet3.csv' # 這里是已經(jīng)利用SMOTE進(jìn)行過(guò)預(yù)處理的數(shù)據(jù)集 max_depth = 15 # 調(diào)參(自己修改) #決策樹(shù)深度不能太深,不然容易導(dǎo)致過(guò)擬合 min_size = 1 sample_ratio = 1 trees_num = 20 feature_ratio = 0.3 # 盡可能小,但是要保證 int(self.feature_ratio * (len(train[0])-1)) 大于1 same_value = 20 # 向量?jī)?nèi)積的差(小于此值認(rèn)為相似) same_rate = 0.82 # 樹(shù)的相似度(大于此值認(rèn)為相似) # 加載數(shù)據(jù) dataset,features = load_csv(filename) traindata,testdata = split_train_test(dataset, feature_ratio) # 優(yōu)化1-獲取最優(yōu)深度 # max_depth = getBestDepth(min_size, sample_ratio, trees_num, feature_ratio, traindata, testdata) # print('max_depth is ',max_depth) # 初始化隨機(jī)森林 myRF = randomForest(trees_num, max_depth, min_size, sample_ratio, feature_ratio) # 生成隨機(jī)森林 myRF.build_randomforest(traindata) print('Tree_number: ', myRF.trees.__len__()) acc = myRF.accuracy_metric(testdata[:-1]) print('傳統(tǒng)RF模型準(zhǔn)確率:',acc,'%') # 畫出某棵樹(shù)用以可視化觀察(這里是第一棵樹(shù)) # plotTree.creatPlot(myRF.trees[0], features) # 計(jì)算森林中每棵樹(shù)的AUC auc_list = caculateAUC_1.caculateRFAUC(testdata,myRF.trees) # 畫出每棵樹(shù)的auc――柱狀圖 # plotTree.plotAUCbar(auc_list.__len__(),auc_list) # 選取AUC高的決策數(shù)形成新的森林(auc優(yōu)化) newTempForest = auc_optimization(auc_list,trees_num,myRF.trees) # 相似度優(yōu)化 myRF.trees=similarity_optimization(newTempForest, same_value, same_rate) print('優(yōu)化后Tree_number: ', myRF.trees.__len__()) # 測(cè)試評(píng)估 acc = myRF.accuracy_metric(testdata[:-1]) # print('優(yōu)化后模型準(zhǔn)確率:', acc, '%') print('myRF1_模型準(zhǔn)確率:', acc, '%') # 畫出某棵樹(shù)用以可視化觀察(這里是第一棵樹(shù)) # plotTree.creatPlot(myRF.trees[0], features) # 計(jì)算森林中每棵樹(shù)的AUC auc_list = caculateAUC_1.caculateRFAUC(testdata, myRF.trees) # 畫出每棵樹(shù)的auc――柱狀圖 plotTree.plotAUCbar(auc_list.__len__(), auc_list) end = time.clock() print('The end!') print(end-start)
以上就是本文的全部?jī)?nèi)容,希望對(duì)大家的學(xué)習(xí)有所幫助,也希望大家多多支持腳本之家。
更多文章、技術(shù)交流、商務(wù)合作、聯(lián)系博主
微信掃碼或搜索:z360901061
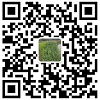
微信掃一掃加我為好友
QQ號(hào)聯(lián)系: 360901061
您的支持是博主寫作最大的動(dòng)力,如果您喜歡我的文章,感覺(jué)我的文章對(duì)您有幫助,請(qǐng)用微信掃描下面二維碼支持博主2元、5元、10元、20元等您想捐的金額吧,狠狠點(diǎn)擊下面給點(diǎn)支持吧,站長(zhǎng)非常感激您!手機(jī)微信長(zhǎng)按不能支付解決辦法:請(qǐng)將微信支付二維碼保存到相冊(cè),切換到微信,然后點(diǎn)擊微信右上角掃一掃功能,選擇支付二維碼完成支付。
【本文對(duì)您有幫助就好】元
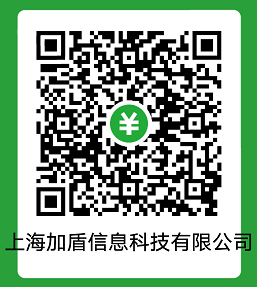