本文實(shí)例為大家分享了python多進(jìn)程讀圖提取特征存npy的具體代碼,供大家參考,具體內(nèi)容如下
import multiprocessing import os, time, random import numpy as np import cv2 import os import sys from time import ctime import tensorflow as tf image_dir = r"D:/sxl/處理圖片/漢字分類/train10/" #圖像文件夾路徑 data_type = 'test' save_path = r'E:/sxl_Programs/Python/CNN/npy/' #存儲(chǔ)路徑 data_name = 'Img10' #npy文件名 char_set = np.array(os.listdir(image_dir)) #文件夾名稱列表 np.save(save_path+'ImgShuZi10.npy',char_set) #文件夾名稱列表 char_set_n = len(char_set) #文件夾列表長(zhǎng)度 read_process_n = 1 #進(jìn)程數(shù) repate_n = 4 #隨機(jī)移動(dòng)次數(shù) data_size = 1000000 #1個(gè)npy大小 shuffled = True #是否打亂 #可以讀取帶中文路徑的圖 def cv_imread(file_path,type=0): cv_img=cv2.imdecode(np.fromfile(file_path,dtype=np.uint8),-1) # print(file_path) # print(cv_img.shape) # print(len(cv_img.shape)) if(type==0): if(len(cv_img.shape)==3): cv_img = cv2.cvtColor(cv_img, cv2.COLOR_BGR2GRAY) return cv_img #多個(gè)數(shù)組按同一規(guī)則打亂數(shù)據(jù) def ShuffledData(features,labels): ''' @description:隨機(jī)打亂數(shù)據(jù)與標(biāo)簽,但保持?jǐn)?shù)據(jù)與標(biāo)簽一一對(duì)應(yīng) ''' permutation = np.random.permutation(features.shape[0]) shuffled_features = features[permutation,:] #多維 shuffled_labels = labels[permutation] #1維 return shuffled_features,shuffled_labels #函數(shù)功能:簡(jiǎn)單網(wǎng)格 #函數(shù)要求:1.無(wú)關(guān)圖像大小;2.輸入圖像默認(rèn)為灰度圖;3.參數(shù)只有輸入圖像 #返回?cái)?shù)據(jù):1x64*64維特征 def GetFeature(image): #圖像大小歸一化 image = cv2.resize(image,(64,64)) img_h = image.shape[0] img_w = image.shape[1] #定義特征向量 feature = np.zeros(img_h*img_w,dtype=np.int16) for h in range(img_h): for w in range(img_w): feature[h*img_h+w] = image[h,w] return feature # 寫數(shù)據(jù)進(jìn)程執(zhí)行的代碼: def read_image_to_queue(queue): print('Process to write: %s' % os.getpid()) for j,dirname in enumerate(char_set): # dirname 是文件夾名稱 label = np.where(char_set==dirname)[0][0] #文件夾名稱對(duì)應(yīng)的下標(biāo)序號(hào) print('序號(hào):'+str(j),'讀 '+dirname+' 文件夾...時(shí)間:',ctime() ) for parent,_,filenames in os.walk(os.path.join(image_dir,dirname)): for filename in filenames: if(filename[-4:]!='.jpg'): continue image = cv_imread(os.path.join(parent,filename),0) # cv2.imshow(dirname,image) # cv2.waitKey(0) queue.put((image,label)) for i in range(read_process_n): queue.put((None,-1)) print('讀圖結(jié)束!') return True # 讀數(shù)據(jù)進(jìn)程執(zhí)行的代碼: def extract_feature(queue,lock,count): ''' @description:從隊(duì)列中取出圖片進(jìn)行特征提取 @queue:先進(jìn)先出隊(duì)列 lock:鎖,在計(jì)數(shù)時(shí)上鎖,防止沖突 count:計(jì)數(shù) ''' print('Process %s start reading...' % os.getpid()) global data_n features = [] #存放提取到的特征 labels = [] #存放標(biāo)簽 flag = True #標(biāo)志著進(jìn)程是否結(jié)束 while flag: image,label = queue.get() #從隊(duì)列中獲取圖像和標(biāo)簽 if len(features) >= data_size or label == -1: #特征數(shù)組的長(zhǎng)度大于指定長(zhǎng)度,則開始存儲(chǔ) array_features = np.array(features) #轉(zhuǎn)換成數(shù)組 array_labels = np.array(labels) array_features,array_labels = ShuffledData(array_features,array_labels) #打亂數(shù)據(jù) lock.acquire() # 鎖開始 # 拆分?jǐn)?shù)據(jù)為訓(xùn)練集,測(cè)試集 split_x = int(array_features.shape[0] * 0.8) train_data, test_data = np.split(array_features, [split_x], axis=0) # 拆分特征數(shù)據(jù)集 train_labels, test_labels = np.split(array_labels, [split_x], axis=0) # 拆分標(biāo)簽數(shù)據(jù)集 count.value += 1 #下標(biāo)計(jì)數(shù)加1 str_features_name_train = data_name+'_features_train_'+str(count.value)+'.npy' str_labels_name_train = data_name+'_labels_train_'+str(count.value)+'.npy' str_features_name_test = data_name+'_features_test_'+str(count.value)+'.npy' str_labels_name_test = data_name+'_labels_test_'+str(count.value)+'.npy' lock.release() # 鎖釋放 np.save(save_path+str_features_name_train,train_data) np.save(save_path+str_labels_name_train,train_labels) np.save(save_path+str_features_name_test,test_data) np.save(save_path+str_labels_name_test,test_labels) print(os.getpid(),'save:',str_features_name_train) print(os.getpid(),'save:',str_labels_name_train) print(os.getpid(),'save:',str_features_name_test) print(os.getpid(),'save:',str_labels_name_test) features.clear() labels.clear() if label == -1: break # 獲取特征向量,傳入灰度圖 feature = GetFeature(image) features.append(feature) labels.append(label) # # 隨機(jī)移動(dòng)4次 # for itime in range(repate_n): # rMovedImage = randomMoveImage(image) # feature = SimpleGridFeature(rMovedImage) # 簡(jiǎn)單網(wǎng)格 # features.append(feature) # labels.append(label) print('Process %s is done!' % os.getpid()) if __name__=='__main__': time_start = time.time() # 開始計(jì)時(shí) # 父進(jìn)程創(chuàng)建Queue,并傳給各個(gè)子進(jìn)程: image_queue = multiprocessing.Queue(maxsize=1000) #隊(duì)列 lock = multiprocessing.Lock() #鎖 count = multiprocessing.Value('i',0) #計(jì)數(shù) #將圖寫入隊(duì)列進(jìn)程 write_sub_process = multiprocessing.Process(target=read_image_to_queue, args=(image_queue,)) read_sub_processes = [] #讀圖子線程 for i in range(read_process_n): read_sub_processes.append( multiprocessing.Process(target=extract_feature, args=(image_queue,lock,count)) ) # 啟動(dòng)子進(jìn)程pw,寫入: write_sub_process.start() # 啟動(dòng)子進(jìn)程pr,讀取: for p in read_sub_processes: p.start() # 等待進(jìn)程結(jié)束: write_sub_process.join() for p in read_sub_processes: p.join() time_end=time.time() time_h=(time_end-time_start)/3600 print('用時(shí):%.6f 小時(shí)'% time_h) print ("讀圖提取特征存npy,運(yùn)行結(jié)束!")
以上就是本文的全部?jī)?nèi)容,希望對(duì)大家的學(xué)習(xí)有所幫助,也希望大家多多支持腳本之家。
更多文章、技術(shù)交流、商務(wù)合作、聯(lián)系博主
微信掃碼或搜索:z360901061
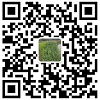
微信掃一掃加我為好友
QQ號(hào)聯(lián)系: 360901061
您的支持是博主寫作最大的動(dòng)力,如果您喜歡我的文章,感覺我的文章對(duì)您有幫助,請(qǐng)用微信掃描下面二維碼支持博主2元、5元、10元、20元等您想捐的金額吧,狠狠點(diǎn)擊下面給點(diǎn)支持吧,站長(zhǎng)非常感激您!手機(jī)微信長(zhǎng)按不能支付解決辦法:請(qǐng)將微信支付二維碼保存到相冊(cè),切換到微信,然后點(diǎn)擊微信右上角掃一掃功能,選擇支付二維碼完成支付。
【本文對(duì)您有幫助就好】元
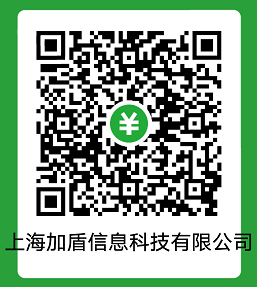