在之前學(xué)習(xí)的RDD和DataFrame數(shù)據(jù)集主要處理的是離線數(shù)據(jù),隨著時代發(fā)展進步,我們會發(fā)現(xiàn)越來越多數(shù)據(jù)是在源源不斷發(fā)回到數(shù)據(jù)中心,同時需要立刻響應(yīng)給用戶,這樣的情況我們就會用到實時處理,常用的場景有實時顯示某商場一小時人流密度、實時顯示當天火車站人口總數(shù)等等。接下來從實時數(shù)據(jù)源說起,實時數(shù)據(jù)源主要有:
- File Source
- Socket Source
- Flume Source
- Kafka Source
File Source指的是文件作為數(shù)據(jù)來源,常用的有本地文件file和分布式系統(tǒng)hdfs,這邊以本地文件來說明,實現(xiàn)代碼如下
from pyspark import SparkContext
from pyspark.streaming import StreamingContext
if __name__ == '__main__':
sc = SparkContext(appName="inputSourceStreaming", master="local[*]")
# 第二個參數(shù)指統(tǒng)計多長時間的數(shù)據(jù)
ssc = StreamingContext(sc, 5)
lines = ssc.textFileStream("file:///home/llh/data/streaming")
counts = lines.flatMap(lambda line: line.split(" ")).map(lambda x: (x, 1)).reduceByKey(lambda a,b: a+b)
counts.pprint()
# -------------------------------------------
# Time: 2019-07-31 18:11:55
# -------------------------------------------
# ('hong', 2)
# ('zhang', 2)
# ('li', 2)
# ('san', 2)
# ('wang', 2)
ssc.start()
ssc.awaitTermination()
然后不斷向/home/llh/data/streaming/目錄下拷貝文件,結(jié)果如上面注釋所示
Socket Source指網(wǎng)絡(luò)套接字作為數(shù)據(jù)來源,用命令nc模擬網(wǎng)絡(luò)發(fā)送信息,實現(xiàn)代碼如下
from pyspark import SparkContext
from pyspark.streaming import StreamingContext
if __name__ == '__main__':
sc = SparkContext(appName="inputSourceStreaming", master="local[*]")
# 第二個參數(shù)指統(tǒng)計多長時間的數(shù)據(jù)
ssc = StreamingContext(sc, 5)
lines = ssc.socketTextStream("localhost", 9999)
counts = lines.flatMap(lambda line: line.split(" ")).map(lambda x: (x, 1)).reduceByKey(lambda a,b: a+b)
counts.pprint()
# -------------------------------------------
# Time: 2019-07-31 18: 43:25
# -------------------------------------------
# ('hadoop', 1)
# ('spark', 1)
ssc.start()
ssc.awaitTermination()
命令端執(zhí)行~$ nc -lk 9999
hadoop spark
之后運行代碼即可
Flume是一個高可用海量收集日志系統(tǒng),因此可作為數(shù)據(jù)來源,實現(xiàn)代碼如下
from pyspark import SparkContext
from pyspark.streaming import StreamingContext
from pyspark.streaming.flume import FlumeUtils
if __name__ == '__main__':
sc = SparkContext(appName="inputSourceStreaming", master="local[*]")
# 第二個參數(shù)指統(tǒng)計多長時間的數(shù)據(jù)
ssc = StreamingContext(sc, 5)
lines = FlumeUtils.createStream("localhost", 34545)
counts = lines.flatMap(lambda line: line.split(" ")).map(lambda x: (x, 1)).reduceByKey(lambda a,b: a+b)
counts.pprint()
ssc.start()
ssc.awaitTermination()
Kafka是一款分布式消息隊列,常作為中間件用于傳輸,隔離,Kafka是以上四種里面實際開發(fā)最常用的流式數(shù)據(jù)來源,一樣實現(xiàn)代碼如下
from pyspark import SparkContext
from pyspark.streaming import StreamingContext
from pyspark.streaming.kafka import KafkaUtils
if __name__ == '__main__':
sc = SparkContext(appName="inputSourceStreaming", master="local[*]")
# 第二個參數(shù)指統(tǒng)計多長時間的數(shù)據(jù)
ssc = StreamingContext(sc, 5)
kvs = KafkaUtils.createDirectStream(ssc, "topic-name", "localhost:9092")
lines = kvs.map(lambda x: x[1])
counts = lines.flatMap(lambda line: line.split(" ")).map(lambda x: (x, 1)).reduceByKey(lambda a,b: a+b)
counts.pprint()
ssc.start()
ssc.awaitTermination()
好了,以上就是實時處理主要數(shù)據(jù)來源,第四種最為重要必須掌握。
?
Spark學(xué)習(xí)目錄:
- Spark學(xué)習(xí)實例1(Python):單詞統(tǒng)計 Word Count
- Spark學(xué)習(xí)實例2(Python):加載數(shù)據(jù)源Load Data Source
- Spark學(xué)習(xí)實例3(Python):保存數(shù)據(jù)Save Data
- Spark學(xué)習(xí)實例4(Python):RDD轉(zhuǎn)換 Transformations
- Spark學(xué)習(xí)實例5(Python):RDD執(zhí)行 Actions
- Spark學(xué)習(xí)實例6(Python):共享變量Shared Variables
- Spark學(xué)習(xí)實例7(Python):RDD、DataFrame、DataSet相互轉(zhuǎn)換
- Spark學(xué)習(xí)實例8(Python):輸入源實時處理 Input Sources Streaming
- Spark學(xué)習(xí)實例9(Python):窗口操作 Window Operations
更多文章、技術(shù)交流、商務(wù)合作、聯(lián)系博主
微信掃碼或搜索:z360901061
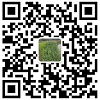
微信掃一掃加我為好友
QQ號聯(lián)系: 360901061
您的支持是博主寫作最大的動力,如果您喜歡我的文章,感覺我的文章對您有幫助,請用微信掃描下面二維碼支持博主2元、5元、10元、20元等您想捐的金額吧,狠狠點擊下面給點支持吧,站長非常感激您!手機微信長按不能支付解決辦法:請將微信支付二維碼保存到相冊,切換到微信,然后點擊微信右上角掃一掃功能,選擇支付二維碼完成支付。
【本文對您有幫助就好】元
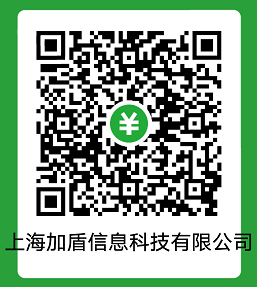