本文python代碼實現的是最小二乘法線性擬合,并且包含自己造的輪子與別人造的輪子的結果比較。
問題:對直線附近的帶有噪聲的數據進行線性擬合,最終求出w,b的估計值。
最小二乘法基本思想是使得樣本方差最小。
代碼中self_func()函數為自定義擬合函數,skl_func()為調用scikit-learn中線性模塊的函數。
import numpy as np import matplotlib.pyplot as plt from sklearn.linear_model import LinearRegression n = 101 x = np.linspace(0,10,n) noise = np.random.randn(n) y = 2.5 * x + 0.8 + 2.0 * noise def self_func(steps=100, alpha=0.01): w = 0.5 b = 0 alpha = 0.01 for i in range(steps): y_hat = w*x + b dy = 2.0*(y_hat - y) dw = dy*x db = dy w = w - alpha*np.sum(dw)/n b = b - alpha*np.sum(db)/n e = np.sum((y_hat-y)**2)/n #print (i,'W=',w,'\tb=',b,'\te=',e) print ('self_func:\tW =',w,'\n\tb =',b) plt.scatter(x,y) plt.plot(np.arange(0,10,1), w*np.arange(0,10,1) + b, color = 'r', marker = 'o', label = 'self_func(steps='+str(steps)+', alpha='+str(alpha)+')') def skl_func(): lr = LinearRegression() lr.fit(x.reshape(-1,1),y) y_hat = lr.predict(np.arange(0,10,0.75).reshape(-1,1)) print('skl_fun:\tW = %f\n\tb = %f'%(lr.coef_,lr.intercept_)) plt.plot(np.arange(0,10,0.75), y_hat, color = 'g', marker = 'x', label = 'skl_func') self_func(10000) skl_func() plt.legend(loc='upper left') plt.show()
結果:
self_func:? W = 2.5648753825503197 ????b = 0.24527830841237772
skl_fun:?????W = 2.564875??????????????????????????? ?b = 0.245278
以上就是本文的全部內容,希望對大家的學習有所幫助,也希望大家多多支持腳本之家。
更多文章、技術交流、商務合作、聯系博主
微信掃碼或搜索:z360901061
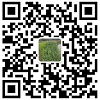
微信掃一掃加我為好友
QQ號聯系: 360901061
您的支持是博主寫作最大的動力,如果您喜歡我的文章,感覺我的文章對您有幫助,請用微信掃描下面二維碼支持博主2元、5元、10元、20元等您想捐的金額吧,狠狠點擊下面給點支持吧,站長非常感激您!手機微信長按不能支付解決辦法:請將微信支付二維碼保存到相冊,切換到微信,然后點擊微信右上角掃一掃功能,選擇支付二維碼完成支付。
【本文對您有幫助就好】元
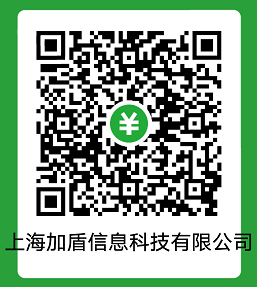