Apriori算法原理: http://blog.csdn.net/kingzone_2008/article/details/8183768
?
import java.util.HashMap; import java.util.HashSet; import java.util.Iterator; import java.util.Map; import java.util.Set; import java.util.TreeMap; /** * <B>關聯規則挖掘:Apriori算法</B> * * <P>按照Apriori算法的基本思想來實現 * * @author king * @since 2013/06/27 * */ public class Apriori { private Map<Integer, Set<String>> txDatabase; // 事務數據庫 private Float minSup; // 最小支持度 private Float minConf; // 最小置信度 private Integer txDatabaseCount; // 事務數據庫中的事務數 private Map<Integer, Set<Set<String>>> freqItemSet; // 頻繁項集集合 private Map<Set<String>, Set<Set<String>>> assiciationRules; // 頻繁關聯規則集合 public Apriori( Map<Integer, Set<String>> txDatabase, Float minSup, Float minConf) { this.txDatabase = txDatabase; this.minSup = minSup; this.minConf = minConf; this.txDatabaseCount = this.txDatabase.size(); freqItemSet = new TreeMap<Integer, Set<Set<String>>>(); assiciationRules = new HashMap<Set<String>, Set<Set<String>>>(); } /** * 掃描事務數據庫,計算頻繁1-項集 * @return */ public Map<Set<String>, Float> getFreq1ItemSet() { Map<Set<String>, Float> freq1ItemSetMap = new HashMap<Set<String>, Float>(); Map<Set<String>, Integer> candFreq1ItemSet = this.getCandFreq1ItemSet(); Iterator<Map.Entry<Set<String>, Integer>> it = candFreq1ItemSet.entrySet().iterator(); while(it.hasNext()) { Map.Entry<Set<String>, Integer> entry = it.next(); // 計算支持度 Float supported = new Float(entry.getValue().toString())/new Float(txDatabaseCount); if(supported>=minSup) { freq1ItemSetMap.put(entry.getKey(), supported); } } return freq1ItemSetMap; } /** * 計算候選頻繁1-項集 * @return */ public Map<Set<String>, Integer> getCandFreq1ItemSet() { Map<Set<String>, Integer> candFreq1ItemSetMap = new HashMap<Set<String>, Integer>(); Iterator<Map.Entry<Integer, Set<String>>> it = txDatabase.entrySet().iterator(); // 統計支持數,生成候選頻繁1-項集 while(it.hasNext()) { Map.Entry<Integer, Set<String>> entry = it.next(); Set<String> itemSet = entry.getValue(); for(String item : itemSet) { Set<String> key = new HashSet<String>(); key.add(item.trim()); if(!candFreq1ItemSetMap.containsKey(key)) { Integer value = 1; candFreq1ItemSetMap.put(key, value); } else { Integer value = 1+candFreq1ItemSetMap.get(key); candFreq1ItemSetMap.put(key, value); } } } return candFreq1ItemSetMap; } /** * 根據頻繁(k-1)-項集計算候選頻繁k-項集 * * @param m 其中m=k-1 * @param freqMItemSet 頻繁(k-1)-項集 * @return */ public Set<Set<String>> aprioriGen(int m, Set<Set<String>> freqMItemSet) { Set<Set<String>> candFreqKItemSet = new HashSet<Set<String>>(); Iterator<Set<String>> it = freqMItemSet.iterator(); Set<String> originalItemSet = null; while(it.hasNext()) { originalItemSet = it.next(); Iterator<Set<String>> itr = this.getIterator(originalItemSet, freqMItemSet); while(itr.hasNext()) { Set<String> identicalSet = new HashSet<String>(); // 兩個項集相同元素的集合(集合的交運算) identicalSet.addAll(originalItemSet); Set<String> set = itr.next(); identicalSet.retainAll(set); // identicalSet中剩下的元素是identicalSet與set集合中公有的元素 if(identicalSet.size() == m-1) { // (k-1)-項集中k-2個相同 Set<String> differentSet = new HashSet<String>(); // 兩個項集不同元素的集合(集合的差運算) differentSet.addAll(originalItemSet); differentSet.removeAll(set); // 因為有k-2個相同,則differentSet中一定剩下一個元素,即differentSet大小為1 differentSet.addAll(set); // 構造候選k-項集的一個元素(set大小為k-1,differentSet大小為k) if(!this.has_infrequent_subset(differentSet, freqMItemSet)) candFreqKItemSet.add(differentSet); // 加入候選k-項集集合 } } } return candFreqKItemSet; } /** * 使用先驗知識,剪枝。若候選k項集中存在k-1項子集不是頻繁k-1項集,則刪除該候選k項集 * @param candKItemSet * @param freqMItemSet * @return */ private boolean has_infrequent_subset(Set<String> candKItemSet, Set<Set<String>> freqMItemSet) { Set<String> tempSet = new HashSet<String>(); tempSet.addAll(candKItemSet); Iterator<String> itItem = candKItemSet.iterator(); while(itItem.hasNext()) { String item = itItem.next(); tempSet.remove(item);// 該候選去掉一項后變為k-1項集 if(!freqMItemSet.contains(tempSet))// 判斷k-1項集是否是頻繁項集 return true; tempSet.add(item);// 恢復 } return false; } /** * 根據一個頻繁k-項集的元素(集合),獲取到頻繁k-項集的從該元素開始的迭代器實例 * @param itemSet * @param freqKItemSet 頻繁k-項集 * @return */ private Iterator<Set<String>> getIterator(Set<String> itemSet, Set<Set<String>> freqKItemSet) { Iterator<Set<String>> it = freqKItemSet.iterator(); while(it.hasNext()) { if(itemSet.equals(it.next())) { break; } } return it; } /** * 根據頻繁(k-1)-項集,調用aprioriGen方法,計算頻繁k-項集 * * @param k * @param freqMItemSet 頻繁(k-1)-項集 * @return */ public Map<Set<String>, Float> getFreqKItemSet(int k, Set<Set<String>> freqMItemSet) { Map<Set<String>, Integer> candFreqKItemSetMap = new HashMap<Set<String>, Integer>(); // 調用aprioriGen方法,得到候選頻繁k-項集 Set<Set<String>> candFreqKItemSet = this.aprioriGen(k-1, freqMItemSet); // 掃描事務數據庫 Iterator<Map.Entry<Integer, Set<String>>> it = txDatabase.entrySet().iterator(); // 統計支持數 while(it.hasNext()) { Map.Entry<Integer, Set<String>> entry = it.next(); Iterator<Set<String>> kit = candFreqKItemSet.iterator(); while(kit.hasNext()) { Set<String> kSet = kit.next(); Set<String> set = new HashSet<String>(); set.addAll(kSet); set.removeAll(entry.getValue()); // 候選頻繁k-項集與事務數據庫中元素做差運算 if(set.isEmpty()) { // 如果拷貝set為空,支持數加1 if(candFreqKItemSetMap.get(kSet) == null) { Integer value = 1; candFreqKItemSetMap.put(kSet, value); } else { Integer value = 1+candFreqKItemSetMap.get(kSet); candFreqKItemSetMap.put(kSet, value); } } } } // 計算支持度,生成頻繁k-項集,并返回 return support(candFreqKItemSetMap); } /** * 根據候選頻繁k-項集,得到頻繁k-項集 * * @param candFreqKItemSetMap 候選k項集(包含支持計數) * @return freqKItemSetMap 頻繁k項集及其支持度(比例) */ public Map<Set<String>, Float> support(Map<Set<String>, Integer> candFreqKItemSetMap) { Map<Set<String>, Float> freqKItemSetMap = new HashMap<Set<String>, Float>(); Iterator<Map.Entry<Set<String>, Integer>> it = candFreqKItemSetMap.entrySet().iterator(); while(it.hasNext()) { Map.Entry<Set<String>, Integer> entry = it.next(); // 計算支持度 Float supportRate = new Float(entry.getValue().toString())/new Float(txDatabaseCount); if(supportRate<minSup) { // 如果不滿足最小支持度,刪除 it.remove(); } else { freqKItemSetMap.put(entry.getKey(), supportRate); } } return freqKItemSetMap; } /** * 挖掘全部頻繁項集 */ public void mineFreqItemSet() { // 計算頻繁1-項集 Set<Set<String>> freqKItemSet = this.getFreq1ItemSet().keySet(); freqItemSet.put(1, freqKItemSet); // 計算頻繁k-項集(k>1) int k = 2; while(true) { Map<Set<String>, Float> freqKItemSetMap = this.getFreqKItemSet(k, freqKItemSet); if(!freqKItemSetMap.isEmpty()) { this.freqItemSet.put(k, freqKItemSetMap.keySet()); freqKItemSet = freqKItemSetMap.keySet(); } else { break; } k++; } } /** * <P>挖掘頻繁關聯規則 * <P>首先挖掘出全部的頻繁項集,在此基礎上挖掘頻繁關聯規則 */ public void mineAssociationRules() { freqItemSet.remove(1); // 刪除頻繁1-項集 Iterator<Map.Entry<Integer, Set<Set<String>>>> it = freqItemSet.entrySet().iterator(); while(it.hasNext()) { Map.Entry<Integer, Set<Set<String>>> entry = it.next(); for(Set<String> itemSet : entry.getValue()) { // 對每個頻繁項集進行關聯規則的挖掘 mine(itemSet); } } } /** * 對從頻繁項集集合freqItemSet中每迭代出一個頻繁項集元素,執行一次關聯規則的挖掘 * @param itemSet 頻繁項集集合freqItemSet中的一個頻繁項集元素 */ public void mine(Set<String> itemSet) { int n = itemSet.size()/2; // 根據集合的對稱性,只需要得到一半的真子集 for(int i=1; i<=n; i++) { // 得到頻繁項集元素itemSet的作為條件的真子集集合 Set<Set<String>> properSubset = ProperSubsetCombination.getProperSubset(i, itemSet); // 對條件的真子集集合中的每個條件項集,獲取到對應的結論項集,從而進一步挖掘頻繁關聯規則 for(Set<String> conditionSet : properSubset) { Set<String> conclusionSet = new HashSet<String>(); conclusionSet.addAll(itemSet); conclusionSet.removeAll(conditionSet); // 刪除條件中存在的頻繁項 confide(conditionSet, conclusionSet); // 調用計算置信度的方法,并且挖掘出頻繁關聯規則 } } } /** * 對得到的一個條件項集和對應的結論項集,計算該關聯規則的支持計數,從而根據置信度判斷是否是頻繁關聯規則 * @param conditionSet 條件頻繁項集 * @param conclusionSet 結論頻繁項集 */ public void confide(Set<String> conditionSet, Set<String> conclusionSet) { // 掃描事務數據庫 Iterator<Map.Entry<Integer, Set<String>>> it = txDatabase.entrySet().iterator(); // 統計關聯規則支持計數 int conditionToConclusionCnt = 0; // 關聯規則(條件項集推出結論項集)計數 int conclusionToConditionCnt = 0; // 關聯規則(結論項集推出條件項集)計數 int supCnt = 0; // 關聯規則支持計數 while(it.hasNext()) { Map.Entry<Integer, Set<String>> entry = it.next(); Set<String> txSet = entry.getValue(); Set<String> set1 = new HashSet<String>(); Set<String> set2 = new HashSet<String>(); set1.addAll(conditionSet); set1.removeAll(txSet); // 集合差運算:set-txSet if(set1.isEmpty()) { // 如果set為空,說明事務數據庫中包含條件頻繁項conditionSet // 計數 conditionToConclusionCnt++; } set2.addAll(conclusionSet); set2.removeAll(txSet); // 集合差運算:set-txSet if(set2.isEmpty()) { // 如果set為空,說明事務數據庫中包含結論頻繁項conclusionSet // 計數 conclusionToConditionCnt++; } if(set1.isEmpty() && set2.isEmpty()) { supCnt++; } } // 計算置信度 Float conditionToConclusionConf = new Float(supCnt)/new Float(conditionToConclusionCnt); if(conditionToConclusionConf>=minConf) { if(assiciationRules.get(conditionSet) == null) { // 如果不存在以該條件頻繁項集為條件的關聯規則 Set<Set<String>> conclusionSetSet = new HashSet<Set<String>>(); conclusionSetSet.add(conclusionSet); assiciationRules.put(conditionSet, conclusionSetSet); } else { assiciationRules.get(conditionSet).add(conclusionSet); } } Float conclusionToConditionConf = new Float(supCnt)/new Float(conclusionToConditionCnt); if(conclusionToConditionConf>=minConf) { if(assiciationRules.get(conclusionSet) == null) { // 如果不存在以該結論頻繁項集為條件的關聯規則 Set<Set<String>> conclusionSetSet = new HashSet<Set<String>>(); conclusionSetSet.add(conditionSet); assiciationRules.put(conclusionSet, conclusionSetSet); } else { assiciationRules.get(conclusionSet).add(conditionSet); } } } /** * 經過挖掘得到的頻繁項集Map * * @return 挖掘得到的頻繁項集集合 */ public Map<Integer, Set<Set<String>>> getFreqItemSet() { return freqItemSet; } /** * 獲取挖掘到的全部的頻繁關聯規則的集合 * @return 頻繁關聯規則集合 */ public Map<Set<String>, Set<Set<String>>> getAssiciationRules() { return assiciationRules; } }
測試類如下:
?
?
import java.io.BufferedReader; import java.io.File; import java.io.FileNotFoundException; import java.io.FileReader; import java.io.IOException; import java.util.HashMap; import java.util.HashSet; import java.util.Map; import java.util.Set; import java.util.TreeSet; import junit.framework.TestCase; /** * <B>Apriori算法測試類</B> * * @author king * @date 2013/07/28 */ public class AprioriTest extends TestCase { private Apriori apriori; private Map<Integer, Set<String>> txDatabase; private Float minSup = new Float("0.50"); private Float minConf = new Float("0.70"); public static void main(String []args) throws Exception { AprioriTest at = new AprioriTest(); at.setUp(); long from = System.currentTimeMillis(); at.testGetFreqItemSet(); long to = System.currentTimeMillis(); System.out.println("耗時:" + (to-from)); } @Override protected void setUp() throws Exception { // create(); // 構造事務數據庫 this.buildData(Integer.MAX_VALUE, "f_faqk_.dat"); apriori = new Apriori(txDatabase, minSup, minConf); } /** * 構造模擬事務數據庫txDatabase */ public void create() { txDatabase = new HashMap<Integer, Set<String>>(); Set<String> set1 = new TreeSet<String>(); set1.add("A"); set1.add("B"); set1.add("C"); set1.add("E"); txDatabase.put(1, set1); Set<String> set2 = new TreeSet<String>(); set2.add("A"); set2.add("B"); set2.add("C"); txDatabase.put(2, set2); Set<String> set3 = new TreeSet<String>(); set3.add("C"); set3.add("D"); txDatabase.put(3, set3); Set<String> set4 = new TreeSet<String>(); set4.add("A"); set4.add("B"); set4.add("E"); txDatabase.put(4, set4); } /** * 構造數據集 * @param fileName 存儲事務數據的文件名 * @param totalcount 獲取的事務數 */ public void buildData(int totalCount, String...fileName) { txDatabase = new HashMap<Integer, Set<String>>(); if(fileName.length !=0){ File file = new File(fileName[0]); int count = 0; try { BufferedReader reader = new BufferedReader(new FileReader(file)); String line; while( (line = reader.readLine()) != null){ String []arr = line.split(" "); Set<String> set = new HashSet<String>(); for(String s : arr) set.add(s); count++; this.txDatabase.put(count, set); if(count >= totalCount) return; } } catch (FileNotFoundException e) { e.printStackTrace(); } catch (IOException e) { e.printStackTrace(); } }else{ } } /** * 測試挖掘頻繁1-項集 */ public void testFreq1ItemSet() { System.out.println("挖掘頻繁1-項集 : " + apriori.getFreq1ItemSet()); } /** * 測試aprioriGen方法,生成候選頻繁項集 */ public void testAprioriGen() { System.out.println( "候選頻繁2-項集 : " + this.apriori.aprioriGen(1, this.apriori.getFreq1ItemSet().keySet()) ); } /** * 測試挖掘頻繁2-項集 */ public void testGetFreq2ItemSet() { System.out.println( "挖掘頻繁2-項集 :" + this.apriori.getFreqKItemSet(2, this.apriori.getFreq1ItemSet().keySet()) ); } /** * 測試挖掘頻繁3-項集 */ public void testGetFreq3ItemSet() { System.out.println( "挖掘頻繁3-項集 :" + this.apriori.getFreqKItemSet( 3, this.apriori.getFreqKItemSet(2, this.apriori.getFreq1ItemSet().keySet()).keySet() ) ); } /** * 測試挖掘全部頻繁項集 */ public void testGetFreqItemSet() { this.apriori.mineFreqItemSet(); // 挖掘頻繁項集 System.out.println("挖掘頻繁項集 :" + this.apriori.getFreqItemSet()); } /** * 測試挖掘全部頻繁關聯規則 */ public void testMineAssociationRules() { this.apriori.mineFreqItemSet(); // 挖掘頻繁項集 this.apriori.mineAssociationRules(); System.out.println("挖掘頻繁關聯規則 :" + this.apriori.getAssiciationRules()); } }
參考:
http://hi.baidu.com/shirdrn/item/5b74a313d55256711009b5d8
?
在此基礎上添加了has_infrequent_subset方法,此方法使用先驗知識進行剪枝,是典型Apriori算法必備的。
?
更多文章、技術交流、商務合作、聯系博主
微信掃碼或搜索:z360901061
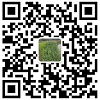
微信掃一掃加我為好友
QQ號聯系: 360901061
您的支持是博主寫作最大的動力,如果您喜歡我的文章,感覺我的文章對您有幫助,請用微信掃描下面二維碼支持博主2元、5元、10元、20元等您想捐的金額吧,狠狠點擊下面給點支持吧,站長非常感激您!手機微信長按不能支付解決辦法:請將微信支付二維碼保存到相冊,切換到微信,然后點擊微信右上角掃一掃功能,選擇支付二維碼完成支付。
【本文對您有幫助就好】元
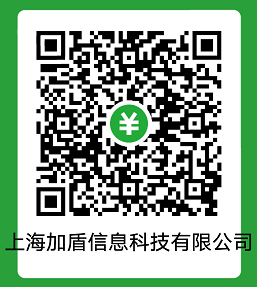